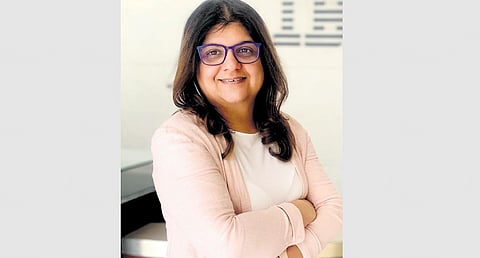
The future with AI and the future of AI are two key questions haunting everyone as we see artificial intelligence (AI) seeping into every aspect of life. In an interaction with Dipak Mondal of New Indian Express, Geeta Gurnani, chief technology officer, IBM India, says Generative AI has made AI very consumable and very easy for everybody.
Excerpt:
Where is AI heading?
We feel AI is at a Netscape moment, what probably Netscape did to the Internet, that it made it very consumable and easy for everyone. I think AI has come to that point, thanks to Generative AI. AI was not new, we were using it. I have been part of the AI journey from 2015 onwards. So, I can say we are in that Netscape moment of AI where people can leverage AI to do and drive a lot more efficiencies in the way we work and operate.I would say it has become very real for enterprises how they leverage and everybody is looking to see how we can leverage in every part of my business to largely drive the efficiency part of it. Revenue is still a second pillar. The first pillar remains cost optimization and efficiency.
Will AI grow bigger than human intelligence?
As a philosophy, we are saying that the purpose of AI will always stay as augmenting human intelligence; it’s never going to replace humans. AI will help free up humans from doing repetitive mundane tasks so that business user can use their business domain knowledge rather than doing some administrative tasks. It is clear that it will impact humans and digital labour, but it will free up individuals to do a more meaningful job, which is where you probably need human intelligence to come in. We do not see AI replacing humans.
Can you elaborate on data governance and its significance in the age of AI?
With my own experience dealing with a lot of enterprises and customers, I would say everybody is aware that data governance is a critical piece. It has become more critical in the Gen AI journey because now you are using the data used to train the models that are tomorrow going to make the decisions. If your data is not right and governed, it will be more like garbage in garbage out.
It will become critical because when you use data outside of your enterprise data, you need to be clear about the sources and what are the copyrights for each of those sources of data. So, data governance will become extremely important because the foundation of AI models is data. If we don’t govern that part, we will land up in a situation where AI will not give us the outcome we would expect.
At what levels can we have governance?
Let’s move from the data to the AI governance part of it. There are five areas where governance needs to ensure that you are leading an ethical AI practice in an organisation. That ethical AI will need five things. First is explainability, which is complete transparency on how the model arrived at a particular decision point. If we don’t know how the decision was made then it is a challenge.
The second area is fairness, which means properly calibrating the training and data so that AI assists in making fair choices and it doesn’t give a biased choice to somebody. Hence, the training data should not be biased. The third is robustness, which indicates that systems need to be robust in terms of security since these are critical training and data systems that people are operating. If we don’t put security guardrails around it, we are at risk of leaking the data or somebody changing the training data itself.
The fourth is transparency, which is designing the model in a way that it supports transparency. At any point in time if I want to know how much bias my model has right now, because there will always be a factor of bias. And the fifth one is privacy, which means you need to be absolutely clear that when you are even using consumer data, you are maintaining their privacy and leveraging their data.
Who should govern data and at what levels should it be governed?
I will say it’s at a very initial stage, but as a client zero, because we also use AI in a lot of our products, we have our own IBM Ethics Board, which is outside of every business unit and is as a central, cross-disciplinary body to support a culture of ethical, responsible and trustworthy AI throughout IBM. Once you source data you need to run some checks and balances to check if there is any hate language or profanity in it.
The ethics board generally stays at a top level in the organisation and the legal teams should also be a part. The most important part is the element of cultural change in the organisation at an enterprise level where everybody needs to be sensitised right from top to bottom on ethical AI and the role everybody plays in it.
Who should AI be explainable to?
Anybody can ask for explainability as an authority. For example, if my loan application gets rejected and it was the basis of the decision of AI, I file a complaint with the bank. The bank should check internally why the application was rejected. It can be initiated by anybody, but there will be a team in every organisation that could be even part of the ethics board itself saying that there is a complaint against decisions made using AI. They can ask for an audit report and all decision points on which this decision was made.
How well prepared are the regulators in respective industry sectors?
I would say it is very hard to comment right now because we have yet to see a real one coming out. I think each one of them right now is working towards it. A lot of organisations have not productionised these use cases, they are experimenting for now. They are doing these use cases where risk is low, which means for internal use cases – for HR, for IT support that are not consumer-facing right now. In the absence of governance, I see that people are not comfortable producing it straight away.
Is data privacy creating constraints in terms of developing those AI models internally?
I would say data is not the blocker here because, in the enterprise context, they largely use their data. But what people are sceptical about is that certain foundation models in GenAI are built on open data available in the public domain. The hesitation is that people do not have a data lineage of that model that they are using from outside. They need this tool to give them complete transparency on where the data in this model was sourced and if it is governed or not. The confidence level is low right now because of these models which are built on public domain data points.
Has there been any kind of talks within the industry to create trust in the data that are used for building AI models?
There have been conversations in the industry for sure because everybody is realising that scaling is not happening as enterprises are not able to scale adequately with people using data in bits and pieces. At an industry collaboration level, open-source communities like Hugging Face are talking about it but I don’t have any formal visibility in terms of what is the road map of industry bodies to come together and solve this.
What is the next stage where AI can help in revenue generation?
It’s a matter of practical application. The domain and applicability remain more on the overall back-office automation and outcome part of it. But a lot of media and content is getting generated by GenAI, and people will certainly use content generation as a key use case to improve customer experience and eventually increase the revenue wallet with customers.