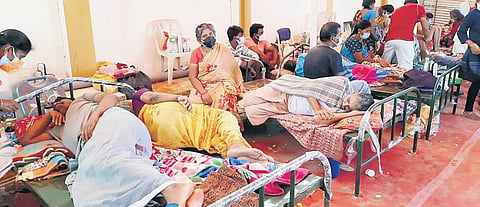
Evidence-based policies and preparedness are crucial to win the war against the Covid-19 pandemic. Mathematical models of infectious diseases play a pivotal role in framing science-informed policies, including lockdown/unlock, hospitalisation, treatment planning, oxygen supply, vaccination, etc. Therefore, the projections of the models should be reliable and account for uncertainties. Though deploying robust and accurate models is very important, feeding necessary and correct data to these models is crucial for reliable predictions. One can argue that the mathematical models/modellers have failed and been proven wrong. Nevertheless, in reality, we fail the mathematical model by feeding incorrect data. Therefore, an initiative to establish a centralised robust data collection and sharing mechanism is needed.
Despite having several models and modellers, a nation relying on a single projection to estimate the complex Covid spread reflects our poor understanding of computational science. For Covid-like pandemic forecasts, we need an ensemble of projections and should avoid over-reliance on a single model. We should encourage researchers to share their forecasts and create an ensemble of predictions like CDC’s predictions in the US. Our preparedness and policies should be based on the ensemble’s prediction.
Computational science is a subject that deals with the data-driven modelling of dynamics and physical processes. Data-driven modelling is a cycle and it consists of several stages:
1) Describing the dynamics by mathematical models using the laws of physics/chemistry/biology or machine learning models using data.
2) Simplification of these complex models with certain assumptions.
3) Identification and fitting of model parameters using either designed experiments or observations.
4) Depending on the complexity of the model, analytical, numerical or computational solutions are obtained.
5) Validation and verification of the model, including stakeholder engagement and feedback.
If the solution needs improvement, the assumptions need to be relooked; the parameters need to be re-estimated; a better numerical scheme has to be employed; and/or the evaluation needs to be improved. Traversing the cycle over and over again makes the computational model more robust, accurate and reliable.
Computational science for epidemiology is a multidisciplinary study that includes epidemiologists, public health experts, healthcare professionals, applied mathematicians, statisticians and computational scientists. The objective of mathematical modelling of infectious diseases is to provide short-term or long-term forecasts for the spread of the disease and is no different from data-driven modelling. Short-term or statistical models are diagnostic ones, where insights are derived from the history or available data. Long-term or dynamical ones are prognostic or predictive, where the projections are made using the insights derived from the statistical models.
The earliest mathematical model of infectious diseases dates back to 1760, when Daniel Bernoulli studied the life expectancy due to vaccination against smallpox. The most popular infectious disease model today is the compartment one developed by Kermack and McKendrick in 1927. Here, the population is divided into three compartments (or homogenous groups): susceptible, infected and recovered. Ordinary differential equations describe the time evolution of the number of people in each homogenous compartment. These equations are coupled to balance the total population. This simple compartment model is known as the SIR model and forms the basis for several variants: SEIR, SAIR, SUTRA, etc. A different approach is used in agent-based models (ABMs), which are essentially stochastic ones for Covid modelling. These ABMs consider an individual or a group as an agent and compute the simultaneous interactions of these multiple agents. After that, probabilistic laws that describe the interaction of agents are specified and used to predict distributions of potential outcomes. Crucially, these models are highly data-hungry and need information about individual agent behaviour.
Though these are robust to model the spread of infectious diseases, Covid modelling poses new challenges and questions. Are the predictions from these models enough to frame timely science-informed policies to revive normalcy in the world? How can we incorporate lockdown/unlock strategies, quarantine rules, seroprevalence surveys, unreported cases, hospitalisation criterion, treatment protocol, vaccination schedules, mutations of viruses, etc., into the model? Among the infected population, don’t we need geographical (country/state/district/zone) information, age of infection, population age and the severity of the disease? These questions necessitate a new generation of pandemic models. More than a century after introducing the SIR model, a multidimensional, partial differential equation model has been proposed recently. This multidimensional model provides the number of infected people with insights into geographical information, the severity of the disease, the age of the infection and the age of the population. Significantly, the severity of the disease is crucial to plan hospitalisation, ICU or oxygen bed needs and treatments.
Improved models, reliable data and ensemble predictions will enable policymakers to frame evidence-based policies that help us tackle Covid and future pandemics in a better way.
Sashikumaar Ganesan and Deepak Subramani
Faculty, Department of Computational and Data Sciences, Indian Institute of Science, Bengaluru
(sashi@iisc.ac.in,
deepakns@iisc.ac.in)