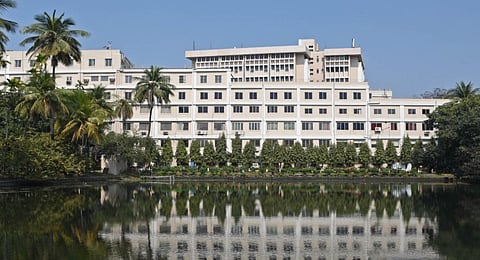
The Indian Statistical Institute is 91 years old. Despite its near-century of existence, numbers from the Indian government's official statistical agency continues to be viewed with skepticism by many. In fact, the latest debate on our archaic statistical framework has ensued just weeks after the country celebrated the National Statistics Day on June 29.
Noted economists from the Left, the Right and the 'Centre' (government) are busy exchanging verbal volleys on how suboptimal methodologies and sampling errors can often lead to a failure to assess actual progress, or the lack of it.
The animosity towards official economic data has reached such a point that all official data, even when valid, is seen as a trick to pat on the government's back.
At the same time, there's no denying that India's statistical methods need a complete overhaul to improve accuracy and regain trust, despite the significant revisions they have undergone in the past decade.
A GLOBAL PHENOMENON
Distrust of official data is not limited to India. Statistical manipulation or statistical crimes occur everywhere, and shockingly, some of them have even claimed lives. Below, The New Indian Express chronicles some of the infamous ones.
The pharmaceutical industry has been second to none when it comes to misrepresenting data. One of the biggest statistical disasters in the healthcare sector dates back to 1996 when Purdue Pharma launched its addictive drug OxyContin in the US, setting off one of the most debilitating health crises in the country and unleashing a wave of opioid addiction that has claimed thousands of lives.
Advertised as a safe, non-addictive drug that was highly effective for pain relief, Purdue’s marketing strategy used an unethical and misleading graph to get FDA approval for the product. It went on to convince doctors that the drug was non-addictive because it stayed in the patient’s blood for longer, helping avoid symptoms of withdrawal. However, it was later found that the graph incorrectly showed the drug's efficacy to spur sales and Purdue was forced to pay a $600 million fine for its criminal actions.
Another famous catastrophe, where statistics played a part, was in 1986, when seven astronauts were killed as their space shuttle 'Challenger' exploded barely 73 seconds into the flight. A subsequent inquiry found a major flaw in statistical analysis by the solid rocket booster engineers, who had excluded flights with zero incidents from their analysis as they felt those flights didn't contribute any relevant information.
However, it was found later that all flights with no incidents were launched at temperatures above 65 degrees, while the Challenger was launched on a cold day when temperature was 39 degrees Fahrenheit. The engineers believed that there was no relationship between launch temperature and the number of O-ring (a mechanical seal preventing gas or liquid leak) incidents on a flight, while the commissioned inquiry concluded that '...O-ring performance would have revealed the correlation of O-ring damage in low temperature.'
While such fatal incidents may have declined over time thanks to advanced statistical methods, what hasn't stopped is the misuse of statistics. Despite the availability of technology and advanced data analysis tools, one can still prove anything through data manipulation. Such mistakes could have been forgiven a few decades ago when statistical methods were still taking shape and survey errors could have been seen as the result of a lack of experience.
Talking of the wilful misinterpretation of data, Darrell Huff's 1954 book How to lie with statistics is a classic in its genre. The 129-pager has numerous examples of the rising trend of data abuse. Sample this:
A 1950 survey found that in Yale University, the class of 1924 had an average income of $25,111 a year. But soon, it was realized that the survey presented a grossly inflated figure, as the sample omitted groups that would have depressed the average. Furthermore, it surveyed only people who bothered to respond and only those that Yale could find.
Then there's Literary Digest's infamous 1936 poll that stood out for its erroneous survey results. The magazine had run a poll on every US election since 1920 and even acquired authoritative status after accurately predicting the 1932 election. However, the subsequent survey saw irreparable cracks. The poll had a biased sample of Digest's 10 million subscribers (largely with a Republican tilt), and forecasted a landslide victory for the Republican Presidential candidate Alf Landon, while the actual election was won by the incumbent Franklin Roosevelt.
ALSO READ | Optics of rankings and data deficits
Darrell took delight in detailing the beauty of lying with statistics. If you are told that the average income in a neighborhood was 10,000 pounds a year, which later dives down to 2,000 pounds a year, that may not be, strictly speaking, not a lie. Both figures may be legitimate and legally arrived at. But the trick was using a different kind of average each time. The 10,000 pounds figure could be the mean, or the arithmetic average of the incomes of all the families in the area. You get it by adding up all the incomes and dividing by the number of families there are. The smaller figure could be the median, and tells you that half the families have over 2,000 pounds a year and half have less.
Cut to the current year and averages continue to be a tricky area. A relevant example is presented by Charles Wheelan in his 2015 book Naked Statistics:
Let's say we want a simple but accurate measure of the economic well-being of citizens and to figure out if the middle class is getting richer, poorer, or just staying in the same place. As Wheelan notes, a reasonable answer -- though by no means the right answer -- would be to calculate the change in per capita income in the country over the course of a generation, or roughly 30 years. Per capita income is a simple average: Total income divided by the size of population. By that measure, average income in the US climbed from $7,787 in 1980 to $26,487 in 2010.
Though it's technically correct, and it may well be far from the truth. For one, the figures aren't adjusted for inflation. The bigger problem is that the average income is not equal to the income of an average citizen. "Per capita income merely takes all of the income earned in the country and divides by the number of people, which tells us absolutely nothing about who is earning how much of that income. Explosive growth in the incomes of the top 1% can raise per capita income significantly without putting any more money in the pockets of the other 99%. The country's average income can go up without helping the average American," he concludes.
Among other major instances where statistics went wrong was Albert Kinsey’s controversial book on Sexual Behavior in the Human Male, published in 1948. The 800-odd page tome was a national best-seller alright, but was criticized and challenged by statisticians for its 'convenience sampling,' prone to bias and inaccuracy. In other words, Kinsey interviewed roughly 5,300 men and 6,000 women in his research, but followed no clear sampling methodology and so all his findings eventually turned out to be junk.
These are only some of the countless cases of misleading statistics used in advertising or by the political class.
However, this is not to say statistics are often wrong or that statistical analysis rarely unveil the truth.
For example, as pointed out by economist Tim Harford in his book How To Make the World Add Up, it was a study by Richard Hill Doll and Austin Bradford in 1954 that established that it was cigarette smoking, not vehicular exhaust, that caused a rise in lung cancer. That was a priceless finding.
Still, one cannot ignore the numerous wisecracks warning us about how data and graphs hide more than they reveal. Therefore, in today's world where data is everywhere, the only way out of bad data can be found in Duffel's profound words: Crooks already know the tricks (to manipulate statistics); honest men must learn them in self-defence.