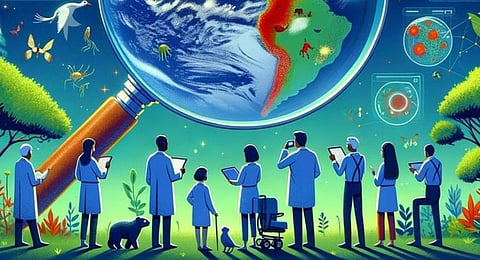
Over the past decade, Artificial Intelligence (AI) has permeated nearly every corner of science: Machine Learning (ML) models have been used to predict protein structures, estimate the fraction of the Amazon rainforest that has been lost to deforestation and even classify faraway galaxies that might be home to exoplanets. But while AI can be used to speed up scientific discovery — helping researchers make predictions about phenomena that may be difficult or costly to study in the real world — it can also lead scientists astray. In the same way that chatbots sometimes “hallucinate”, or make things up. ML models can sometimes present misleading or downright false results.
In a paper published in Science, researchers at the University of California, Berkeley, presented a new statistical technique for safely using the predictions obtained from machine learning models to test scientific hypotheses.
The technique called prediction-powered inference (PPI), uses a small amount of real-world data to correct the output of large, general models — such as AlphaFold, which predicts protein structures — in the context of specific scientific questions. “These models are meant to be general: They can answer many questions, but we don’t know which questions they answer well and which questions they answer badly — and if you use them naively, without knowing which case you’re in, you can get bad answers,” says study author Michael Jordan, the Pehong Chen Distinguished Professor of electrical engineering and computer science and of statistics at UC Berkeley. “With PPI, you’re able to use the model, but correct for possible errors, even when you don’t know the nature of those errors at the outset,” he adds.
The risk of hidden biases
When scientists conduct experiments, they’re not just looking for a single answer — they want to obtain a range of plausible answers. This is done by calculating a “confidence interval”, which, in the simplest case, can be found by repeating an experiment many times and seeing how the results vary. In most science studies, a confidence interval usually refers to a summary or combined statistic, not individual data points. Unfortunately, ML systems focus on individual data points, and thus do not provide scientists with the kinds of uncertainty assessments that they care about. For instance, AlphaFold predicts the structure of a single protein, but it doesn’t provide a notion of confidence for that structure, nor a way to obtain confidence intervals that refer to general properties of proteins.
Scientists may be tempted to use the predictions from AlphaFold as if they were data to compute confidence intervals, ignoring the fact that these predictions. The problem with this approach is that ML systems have many hidden biases that can skew the results. These biases arise, in part, from the data on which they are trained, which are generally existing scientific research that may not have had the same focus as the current study.
Calculating valid confidence intervals
PPI allows scientists to incorporate the predictions from models like AlphaFold without making any assumptions about how the model was built or the data it was trained on. To do this, PPI requires a small amount of data that is unbiased, with respect to the specific hypothesis being investigated, paired with ML predictions corresponding to that data. By bringing these two sources of evidence together, PPI is able to form valid confidence intervals.
For example, the research team applied the PPI technique to algorithms that can pinpoint areas of deforestation in the Amazon using satellite imagery. These models were accurate, overall, when tested individually on regions in the forest; however, when these assessments were combined to estimate deforestation across the entire Amazon, the confidence intervals became highly skewed.
(Source: University of California, Berkeley)